AI Is Improving Medical Monitoring and Follow-Up

Ensuring continuity of care in a clinic or hospital is a nightmare of complexity. Coordinating test results, imaging, medication, and monitoring of vital signs has proven challenging to an industry reliant on ponderous technologies and deficient staffing. When patients are dealing with unfolding health crises and chronic conditions or recovering from procedures at home, managing their care becomes even more complex.
Doctors may miss important findings that can impact patients’ prognosis and treatment — leaving those patients without necessary information on how to make healthcare decisions. Some 97% of available data may go unreviewed per the World Economic Forum. And Electronic Health Records (EHRs) are messy and riddled with errors.
Following up with patients to ensure that they are receiving proper treatment based on the 3% of data that is reviewed constitutes a significant burden on providers.
Even when patients are stable and their cases have received thorough review, they may find that obtaining insights on how to best manage their situations is next to impossible, placing multiple phone calls to overloaded call centers only to spend hours on hold, poring over pages of inscrutable instructions, and attempting to interpret their own results using unreliable home tests and monitors.
Artificial intelligence technologies have shown promise in managing some of the worst inefficiencies in patient follow-up and monitoring. From automated scheduling and chatbots that answer simple questions to review of imaging and test results, a range of AI technologies promise to streamline unwieldy processes for both patients and providers.
These innovations promise to both free up valuable time and increase the likelihood that effective care is delivered. AI chart reviews may detect anomalies that require follow-up and AI review of images may detect early signs of conditions that escape human review.
But, as with other AI technologies, keeping humans in the loop to ensure that algorithmic errors do not result in damage remains challenging. When is a chatbot not enough? And when it isn’t, can a patient actually talk to their provider?
InformationWeek delves into the potential of AI-managed medical monitoring and follow-up, with insights from Angela Adams, CEO of AI imaging follow-up company Inflo Health; and Hamed Akbari, an assistant professor in the Department of Bioengineering at Santa Clara University who works on AI and medical imaging.
Administrative AI
Anyone who has gone through the healthcare system — so, basically everyone — knows how hideous the administrative procedures can be. It’s bad enough trying to schedule a primary care appointment with some clinics. But what about patients who are in recovery from surgery or suffering from debilitating chronic conditions?
AI solutions may smooth out these processes for both the patient and the clinic. AI-assisted platforms offer efficient means of scheduling appointments, refilling prescriptions and getting answers to simple questions about treatment. Patients can simply respond to a text message or fill out a form indicating their needs.
Some 60% of respondents to a 2022 survey preferred intuitive, app-like services from their providers.
Patients may be more inclined to respond to texts or emails generated by AI programs because they can do so on their own time rather than taking a call at an inconvenient moment. They are thus able to provide useful feedback unrelated to their immediate needs — on how they rate their experience with a provider for example — when they might otherwise not be willing to do so.
In the case of anomalous responses — a complication or a dosage problem — a staff member can then follow up with a call or message to address the issue personally. Missed appointments can be flagged, indicating the need for follow-up and also coordinating openings that might be used by other patients who might otherwise need to wait.
More than 70% of patients prefer self-scheduling according to an Experian report. And up to 40% of calls to clinics relate to scheduling. Reduced call volumes can lead to enormous cost savings and free up time for dealing with more exigent issues that require attention and analysis by live medical professionals.
Medication Follow-Up and Adherence
Adherence to medication regimens is essential for many health conditions, both in the wake of acute health events and over time for chronic conditions.
AI programs can both monitor whether patients are taking their medication as prescribed and urge them to do so with programmed notifications. Feedback gathered by these programs can indicate the reasons for non-adherence and help practitioners to devise means of addressing those problems.
Adherence to diabetes management regimens is complicated by lifestyle, socioeconomic status, severity of disease and unique personality factors, for example. AI programs that take these factors into account may assist practitioners and patients in refining protocols so that they are both realistic and effective.
A study that used a smartphone app to remind stroke victims to take their medication and then followed up with blood tests to ensure that they had done so found significant increases in adherence to the drug protocol, resulting in better health outcomes.
AI programs can also use patient data to devise optimal dosing for drugs. Therapeutic drug monitoring has historically been a challenge given the differing reactions of patients to drugs, both alone and in combination, according to their unique physiology.
They can even correlate dosing to the effects of the drugs — a significant advance for conditions in which treatments themselves can have deleterious effects. Chemotherapy drugs, for example, can thus be optimized to maximize effectiveness and minimize side effects.
Monitoring of Chronic Conditions
Using AI to monitor the vital signs of patients suffering from chronic conditions may help to detect anomalies — and indicate adjustments that will stabilize them. Keeping tabs on key indicators of health such as blood pressure, blood sugar, and respiration in a regular fashion can establish a baseline and flag fluctuations that require follow up treatment using both personal and demographic data related to age and sex by comparing it to available data on similar patients.
Remote patient monitoring (RPM) devices, such as blood pressure monitors, pulse oximeters and glucose meters, can be linked to AI programs that analyze the data they collect and draw useful conclusions from it. Education and health literacy levels vary among populations.
Automated summaries can assist patients in understanding the complexities of the information used to determine their status and assume agency in managing their conditions. Even highly educated patients who are already invested in their own care will likely benefit from the efficiency of having their information synthesized in an easily comprehensible manner.
Simplified readouts generated by AI programs can be especially helpful when patients are suffering from multiple conditions — comorbidities — that can make it even more difficult for them to manage their own care and communicate their needs to providers.
Both acute changes and patterns, such as a heart rate that lowers over time, can help providers to assess when interventions such as medication adjustment and even surgery may be necessary.
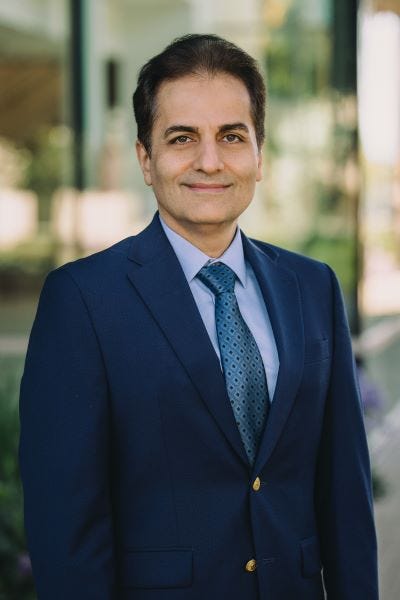
Hamed Akbari, Santa Clara University
Prognosis can be improved if deterioration is detected early. Even in the case of necessary surgery, it can be scheduled prior to an emergent and life-threatening event. In situations where the condition may become life-threatening or terminal, AI may even be able to plot out the likely progression of the disease based on lab findings, allowing for a more realistic approach to treatment and end-of-life planning.
“We have many patients in our studies,” Akbari says. “We know when they passed away. We can determine the length of the survival based on our model.”
Imaging Follow-Up
AI has also shown great promise in augmenting human analysis of radiology findings — X-rays, MRIs, and CT scans, among other technologies. While examination by specialists remains crucial, AI programs now offer increasingly sophisticated means of detecting subtle patterns that may evade even the most skilled radiologists.
An AI analysis of mammograms found that the program was more effective than humans at detecting early signs of breast cancer, for example. Adams relates the story of a friend and colleague whose breast cancer was detected on imaging while she was hospitalized for another condition. However, she was never notified and the cancer fatally metastasized. Adams and her colleagues were horrified that this incidental finding had been missed.
They dug further and found that such incidents were far from uncommon. Even findings that are detected by radiologists do not come to the attention of providers and patients due to time constraints.
“It was astounding to us that nearly 50 to 60% of those follow-ups were just missed,” Adams said. This led to Inflo Health’s mission — reducing missed results and ensuring proper follow-up.
“Non-critical follow-ups definitely need care,” Adams urges. “But findings that are not part of the critical workflow are tossed into a pile.”
Other programs have improved detection of such conditions as pneumonia and appendicitis.
And the identification of novel diseases, such as COVID-19, may also be improved by AI image examination. Radiologists may not be as familiar with the presentation of new diseases on imagery. Rapid identification of patterns in the progression of a new disease using AI programs may be able to assist in diagnosis.
Interpretation of microscopic images has also been improved by AI, allowing for quicker identification of pathogens in samples taken from patients.
The increased follow-up rates can be substantial — Inflo Health’s partnership with the East Alabama Medical Center resulted in a 74% increase in follow ups on lung nodules detected by their technology in radiology reports while reducing the time it took to flag the findings by 95%.
A study on detection of aortic aneurysms found that detection of additional complications was increased by 80% using an AI program. While those complications may have eventually been discovered by human radiologists, the research found that reporting time was reduced by 63%. Other research suggests that AI-assisted scheduling follow-up has improved detection of aneurysm complications. Another project discovered that an AI-enhanced workflow significantly improved follow-up by patients diagnosed with a diabetes-related eye condition.
AI programs can also simplify complex arrays of imagery. “AI can uncover patterns and relationships in imaging data that are not visible to the human eye. You can come up with one map that shows multiple MRI sequences. So instead of looking at five or six different MRI sequences, you just look at one,” Akbari says. And by analyzing large databases of images and the notes that accompany them, these programs can detect early signs of pathology and thus facilitate earlier, more effective treatment.
Such results suggest that collaboration between humans and AI may provide benefits to both patients and the institutions that serve them. Integration into the actual care of the patient is key. If a problem is flagged by an AI program and nothing happens, the finding cannot be acted upon. Adams is insistent that both the patient and provider must be notified when AI programs pick up a finding that has been missed.
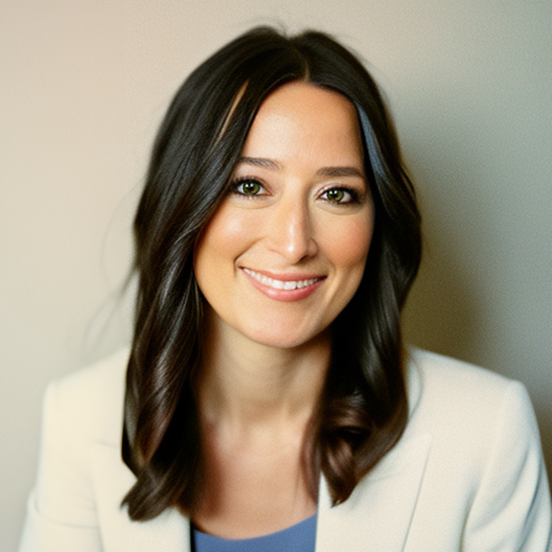
Angela Adams, Inflo Health
“We didn’t just focus on the math and the AI problem,” she says. “We focused on taking that information that we identified and making sure that it worked within the clinical workflow.”
Surgical and Hospitalization Follow-Up
Once a condition has been diagnosed and treated, an additional array of issues emerges. In addition to coordinating appointments to assess progress, at-home care needs to be tracked.
Post-surgical patients are likely to have numerous questions about how to monitor their conditions and ensure that their recovery is proceeding as predicted. This can result in time-consuming phone calls and emails for both patient and provider. Patients are often provided with packets of confusing information that attempt to guide them through recovery. They are likely to encounter situations that are not explained adequately by these materials. Or they may not receive any directions at all.
Adams points to the challenges of following up on hospital visits. “If you think about how quickly a patient is in and out of the ER, many times the final report doesn’t come back until the patient’s already out. It doesn’t even give an opportunity for the clinical team to talk to the patient,” she notes.
Specifically designed chatbots may be able to handle simpler questions that arise and simplify challenging language that some patients may find difficult to interpret.
While it might seem superficially mundane, AI-generated follow-up calls that ensure appointments in the wake of surgeries or hospitalizations may be hugely beneficial. Rehospitalizations in the wake of health events, planned or unplanned, are an indicator of complications and even mortality. They are also a financial liability for hospitals. Medicare reduces reimbursements if patients suffering from certain conditions are readmitted within 30 days, for example.
Keeping patients on track with their care plans, both at home and in follow-up examinations, can reduce rehospitalization events. Manual phone calls have been shown to be helpful in this regard but are time-consuming for both parties. But even automated calls and surveys can facilitate necessary follow-up and reduce rehospitalization.
AI follow-up must be considered carefully, though. While it may result in efficiencies, some patients will likely be hesitant to direct their questions to automated systems while in a tenuous state. One study found that while AI-managed surgical follow-up calls were useful in collecting data and handling administrative tasks, only 11% of calls handled actual medical consultation.
These systems must be designed to identify the need for conversations and in-person examination rather than serve as a barrier. A system designed for cataract surgery follow up, for example, specifically filters routine questions and concerns from those that might necessitate additional treatment.
Technology that is currently used for daily monitoring of healthy patients may also be useful in monitoring patients with certain conditions. One study was cautiously optimistic about using Apple watches to monitor heart abnormalities in cardiac surgery patients.
Personalization of Treatment
The increased sense of autonomy and control offered by these algorithmic approaches may, paradoxically, have a humanizing approach, making patients feel less like lab rats and more like humans who can engage in their own care.
The use of AI to synthesize both historical and live data about individual patients with general data related to their conditions drawn from research and medical record analysis can give both patients and providers a much clearer picture of how to approach their treatment.
Medical professionals often do not have the time — or inclination — to make the sophisticated calculations required to devise optimum care. And patients often find it challenging to advocate for themselves while dealing with both challenging health problems and masses of unfamiliar information.
AI can detect patterns that neither party would be capable of perceiving independently. Once these patterns are identified, patients and providers can more effectively collaborate on how to proceed — whether that be tinkering with the dosage of medications, pursuing follow up on potentially alarming diagnostic findings, or simply discussing potential lifestyle changes and treatment approaches that might affect long-term prognosis.
“I think the future of AI is in integrated diagnosis and treatment planning,” Akbari says. “Communication between different specialties is very limited.”
“Unless you have technology married with process and people, you’re always going to have failure points,” Adams adds. “I would love to see more healthcare AI vendors focus on a holistic approach. When there’s an AI failure in healthcare, it affects all of us. We need to establish trust with clinicians, and the only way to do that is to establish learning partnerships, where we can iterate and learn.”