The AI Power Paradox
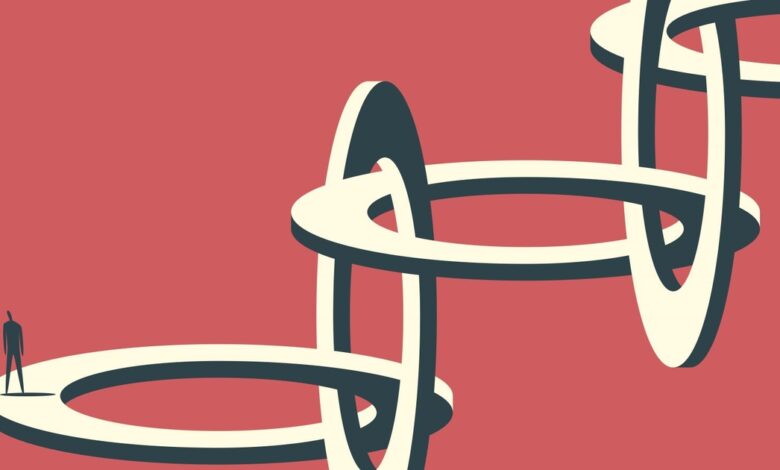
Artificial intelligence is widely touted as our digital savior. If the headlines are to be believed, it will revolutionize everything from how we discover new drugs to how we farm to how we manage our time. Indeed, it has shown great promise in these areas and many others. But these revolutionary advances come at cost — most conspicuously, to our energy grid.
Already strained, the grid will increasingly struggle to keep up with the demands placed on it by training AI models. Other technologies, such as electric vehicles, will further push its limits.
Plans are underway to modernize the world’s aging power infrastructure in an attempt to keep up while moving toward decarbonization — a goal that, worldwide, may cost up to $21 trillion dollars if carbon neutrality is to be achieved by 2050. Perhaps unsurprisingly, AI has also been proposed as a crucial solution to the problem it has, in part, created.
In the United States, the Department of Energy claims that AI will shorten schedules for rolling out new solar and nuclear capacity by 20% and result in savings numbering in the hundreds of billions of dollars. It has announced some $3 billion in grants for research on smart grid technologies, which rely heavily on AI.
Combining machine learning and Internet of Things (IoT) devices, energy providers are collaborating with AI developers to leverage massive amounts of data and streamline the production and provision of electricity to consumers and industry. Improved sensor technology may assist in gathering information on outages and preventing them in the future — outages cost some $150 billion annually in the US alone. And in turn consumers and industry are themselves utilizing AI to make their consumption habits more efficient and even produce their own energy.
“AI -driven software is imperative for the modernization of the power grid. We need to move from steel on the ground to really intelligent software that’s going to enhance resilience,” says Anna Demeo, founder of Climate Tech Strategy Advisors.
Here, InformationWeek investigates the tension between the AI energy drain and the promise that AI has shown in making the grid more sustainable. We include insights from Demeo, Christopher Wellise, vice president of global sustainability at data center provider Equinix and Supratrik Chaudhuri, power and utilities lead at digital consulting company Publicis Sapient.
Power Requirements of AI
AI is a power-intensive technology. Training an AI model takes massive amounts of computing power and that in turn creates a drain on the power grid.
The data center boom partly fueled by uptake of AI will not abate soon, meaning demand will increase exponentially soon. There are nearly 3,000 data centers in the US alone and there will be nearly 5,700 worldwide by the end of this year. The graphics processing units (GPUs) upon which AI training relies take around four times as much power as the central processing units (CPUs) used in traditional computing — and in some AI applications as well. A rack of servers that handles traditional computing tasks requires around 7 kilowatts of energy while a rack handling AI tasks may require up to 100 kilowatts.
“GPUs were originally used in the gaming industry due to their power and their parallel processing capabilities. It was quickly realized that they were ideally suited for things like AI because they generate massive amounts of compute over a short duration,” Wellise explains. “The latest GPUs consume in the neighborhood of 700 watts, compared to the 50 to 85 watts of the standard CPUs.”
Data centers may take as much as 9% of US power supply by 2030, according to the Electric Power Research Institute. Estimates of their drain on global power by 2030 vary — it may be as low as 4%. Wellise says that a range of 6-7% is widely accepted in the industry. This may amount to around 35 gigawatts. A large proportion of this usage will be due to AI and other energy-intensive technologies such as cryptocurrency mining. Around half of the growth in data center load is a result of the AI explosion, according to Lawrence Berkeley National Laboratory.
A single Meta data center in Iowa uses as much power as 7 million laptops every day, according to the company. While large tech companies often claim that they utilize clean energy, if they are purchasing that energy from major power companies, those companies must meet their remaining needs and the needs of their other customers through traditional means — fossil fuels. Reliance on fossil fuel will be exacerbated during periods of low sustainable energy production.
The drain is so substantial that the lifespans of fossil fuel plants scheduled to be decommissioned are being extended to support the increasingly unsustainable demand. Projections of the retirement of coal-fired plants by 2030 have decreased by 40%.
AI is developed in three phases: data engineering, training, and inference. The latter two phases, which organize the data and allow it to be queried respectively, are the most energy intensive. ChatGPT-4, for example, required the same amount of electricity to power 2.5 million homes for a year, according to Wellise.
“As AI progresses, you’re going to see optimization of AI algorithms to begin with,” Demeo says. “We’re going to get better at creating and training these models and then developing more efficient hardware for the data centers themselves.” Until then, though, they will continue to suck power.
By the time AI technologies like ChatGPT come to market, they are far more efficient in terms of energy use. “When you train the model, there’s a lot more computational need and a lot more power usage. When you’re using the model, the need for power is lesser,” Chaudhuri notes. “It’s still higher than your conventional need, but it’s relatively lower.”
But the sheer volume of use itself may pose an equal or even greater challenge to the grid.
Power Distribution By AI
While AI technology may be straining the grid, it is also doing its part to compensate. Some 70% of the grid is now more than 25 years old and AI is providing essential assistance in compensating for this aging infrastructure.
“There’s a joke: If you brought Edison or Tesla back, and showed them the grid in the early 2000s, they would have understood it immediately. In the last 20 years, things have changed dramatically,” Chaudhuri relates.
While the so-called smart grid has not been fully implemented, it has been essential in ensuring that our grizzled network of power plants and transformers and lines remain as functional as possible in the near term.
AI is particularly useful in managing the massive quantities of data involved in distributing energy across the grid, which historically has required a great deal of manual analysis and intervention. Drawing from existing sensors and meters as well as new IoT devices, it can both anticipate need and deliver power from different sources as conditions change in real time — a key benefit as renewable sources contribute more electricity to overall power supply. Because they generate energy through variable natural phenomena like sunlight and wind, availability is intermittent.
“When supply is becoming more and more variable and demand is increasing because of many reasons and the network that connects the two is constrained — it makes for an interesting optimization process,” Chaudhuri notes.
As Demeo points out, the grid has operated on a generation following load model, meaning generation must match demand. Because demand fluctuates, some power must be on standby to meet the need. “We’re in the process of a paradigm shift where we can actually go load following generation as generation is intermittent — the sun and the wind — and we can now manage our load to follow that generation,” she says. “By analyzing these vast data sets, we can predict patterns and predict potential disruptions.”
.jpg?width=700&auto=webp&quality=80&disable=upscale)
Anna Demeo, Climate Tech Strategy Advisors
Directing and storing energy from these sources can be coordinated by AI that integrates information on current and historical weather conditions and consumer demand. It may help in addressing the so-called “duck curve”, for example, which illustrates the disjunct between over-production of solar energy during the day and demand for energy during evening hours, when it is not produced.
Dynamic line rating (DLR) enabled by AI can facilitate higher currents when production of wind or solar energy is high, thus ensuring that more energy gets to consumers more quickly when it is available, by monitoring the conditions that affect the maximum capacity of power lines. Typically, those parameters have been static and thus not optimized for volatile sustainable sources.
And ramping up of production by more carbon-intensive sources and distribution of that energy to make up for the shortfall can be anticipated accordingly. A more sophisticated system of rerouting energy from multiple sources to vulnerable areas of the grid when it is most needed can thus be devised. Doing so automatically at the converter level can reduce the need for centralized controls. The faster reaction time may help to avert outages.
Advanced metering infrastructure (AMI) can assist in providing some of the data necessary to execute these complex tasks. By cataloging when power consumers are most likely to require energy and how much they are likely to need, this technology can further refine how much is produced and how it is allocated.
AI programs can also help power companies to navigate complex regulatory structures, ensuring that all aspects of their operations are in compliance. The DOE has invested $13 million in the VoltAIc initiative, which aims to assist users with siting and permitting, for example. And on the regulatory side, large language models (LLMs) can assist in assessing sentiment analysis from public comment and identifying the parties that need to be involved in decisions about where to install new power infrastructure.
AI and Grid Maintenance
The power grid is a physical structure, a huge network of power plants, transformers, and converters connected by lines leading to the end consumer. As such, it is highly prone to failures — all of these parts are susceptible to mechanical damage. Sometimes, parts just expire.
Storms and other weather events may damage components directly or bring objects such as trees and infrastructure down on them — in some cases resulting in wildfires. Pacific Gas and Electric (PG&E) was assessed some $45 million in penalties due to the fact that its equipment sparked California’s second largest wildfire ever in 2021. The company had previously filed for bankruptcy in 2019 due to tens of billions worth of earlier wildfire related liabilities.
AI has the potential to avert similar catastrophes. Photographs taken by drones can be quickly analyzed by AI programs to ascertain potential problems before they occur, reducing time-consuming manual inspection procedures. Overhanging trees and branches can be removed before they topple onto power lines, for example.
Newly developed AI systems take even broader approaches, analyzing climate risks ranging from snowstorms to fires to winds in order to assess vulnerabilities and correct for them. Sensors and meters installed on these essential parts of the grid can communicate outages to the organizations responsible for fixing them, reducing the time it takes to correct the problems and restore power.
“The grid has a lot more smart devices on it, which are generating a lot more data,” Chaudhuri reports. “Smart meters usually do it once every 15 minutes or so, but there are synchrophasors [which monitor voltage] and other devices that do it several times a second.”
This more proactive mentality will likely be more effective than the scheduled, periodic approach that has until recently been typical of infrastructure maintenance. AI programs can detect performance lags in various components, allowing them to be repaired or replaced before they actually fail and affect power flow. Hours spent on assessing equipment can be reduced, as can costs spent on unnecessary equipment replacement.
“Companies like GE and Siemens can look at transformer failures,” Demeo says. “They can do this with 90% efficiency, which is huge when you think about transformers being these breaking points of the grid.”
AI has applications for maintenance on the generation side, too, Chaudhuri observes. Complex machinery such as steam turbines may take months to repair. AI technology can “disaggregate 10,000 parts to the 10 most critical parts [that] need to be looked at for a certain problem,” he claims.
Managing Data Centers to Reduce AI Power Use
Data center operators love to tout their usage of sustainable energy sources, such as solar and wind. According to an analysis by Goldman Sachs, their claims have some validity — they project that these sources will meet at least 40% of growing data center demand by 2030. But much of the shortfall will be made up by power generated by fossil fuels, particularly natural gas.
Whether the purchase of sustainable energy effectively cancels out the use of more carbon-intensive energy production is highly contested. Critics suggest that data center providers selectively highlight their use of renewable sources while downplaying the consequences of these arrangements for other power users and ignoring their use of fossil fuels when renewables do not meet demand.
Power purchase agreements (PPAs) that match demand on an hourly basis may help to more accurately reflect how much renewable energy a data center is actually using — and drawing on these resources when conditions are optimal for their generation — may reduce competition with other customers.
As Chaudhuri notes, in some regions, tiered rates are being assessed for data centers — those that are performing AI and cryptocurrency functions are charged a higher premium.
Regardless of where the energy comes from, requirements are substantial. And data centers themselves are attempting to reduce them, both by creating efficiencies in their operations and seeking out novel sources of energy.
Implementing more energy-efficient equipment will be crucial. Cooling costs hover around 40% for many data centers, but those that have deployed state-of-the-art technologies such as direct cooling may reduce their costs to only 3% of their total operations. Using direct cooling that delivers liquid to CPUs and GPUs and immersion cooling that places servers in a liquid bath may substantially reduce the level of electricity needed to maintain optimal temperatures for sensitive equipment.
.png?width=700&auto=webp&quality=80&disable=upscale)
Christopher Wellise, Equinix
“Cooling is a large part of what needs to occur within a data center environment, because these GPUs and CPUs generate a lot of heat. One of the ways that we’re able to handle both increased densities around things like the move to GPU is through technologies such as liquid cooling,” Wellise says. “You can optimize for the need for certain types of cooling and the extent to which you need to cool through the use of these digital twins. We were able to actually improve efficiency by 9%.”
The onsite generation of power using solar and wind power or fuel cells using solid oxide or hydrogen technology are among the most promising direct solutions. Other, more experimental, approaches are in the works as well. Google has established a geothermal power plant in Nevada, in partnership with Fervo Energy, for example. The plant supplies the general grid, which Google’s data centers draw from. Other big tech companies are collaborating with nuclear providers to create smaller, localized plants that are aimed at taking pressure off the grid — some might even be sited on data center campuses themselves.
Some, such as Helion, subsidized by Microsoft, hope to harness elusive nuclear fusion technology. By fusing atoms rather than splitting them, as conventional nuclear reactors do, enormous strides in efficiency may be possible. But atomic scientists are highly skeptical as to whether fusion technology will be remotely practicable in the near future.
Wellise notes that AI technologies may also help data centers to manage their energy consumption by monitoring environmental conditions and adjusting use of resources accordingly. “In one of our Frankfurt data centers, we deployed the use of AI to create digital twins where we model data associated with climate parameters,” he explains.
AI can also help tech companies that operate data centers in different areas to allocate their resources according to the availability of renewables. If it is sunny in California and solar energy is available to a data center there, models can ramp up their training at that location and ramp it down in cloudy Virginia, Demeo says. “Just because they’re there doesn’t mean they have to run at full capacity.”
Data center customers, too, can have an impact. They can stipulate that they will only use a data center’s services under certain circumstances. “They will use your data center only until a certain price. Beyond that, they will not use it,” Chaudhuri relates.
Though application of even the most moderate of these technologies is not yet widespread, advocates claim that these experimental setups may eventually be more widely applicable.
“The push for AI might actually complement the push for a more distributed power grid,” Demeo claims. “You can have solar, storage, and smart load management all within a campus for a data center. That can alleviate some of the strain and at the same time upgrade some of the power infrastructure.”
A Distributed Grid
Power generation innovations in data centers are just one component of a nascent distributed grid. Power generation and management will, to an extent, be decentralized as organizations and individual consumers employ technology such as solar panels, wind turbines and batteries for storage — particularly in electric vehicles (EVs).
These power sources may augment power drawn from the grid or even free these “prosumers” from it entirely. In some cases, they may even be able to sell the power they generate back to the grid, further stabilizing it. Home energy management systems (HEMS) will offer guidance on how to manage these resources using data from across the grid, letting users know when generation is peaking and allocating energy at optimal times for different tasks.
“HEMS can make you money when the grid is up, because they can manage when you do things based on time of use rates,” Demeo says. “There’s revenue to be had — not just for the companies that can facilitate the technology. It can also trickle down and be a rebate or refund to individual consumers based on their portion of participation.”
Chaudhuri is a bit more skeptical of energy management in terms of individual consumers, noting that uptake and usage of smart homes has been somewhat tepid. “There’s a bigger ROI on the industry side. If you look at where the demand comes from, residential is far lower,” he says. Still, he thinks that more sophisticated systems may eventually have a market.
.jpg?width=700&auto=webp&quality=80&disable=upscale)
Supratrik Chaudhuri, Publicis Sapient
AI programs can also manage the placement and usage of EV chargers. As more consumers drive EVs, they too will test the grid. By siting them in optimal locations and helping users manage the use of home charging stations, strain on resources can be reduced — ideal charging times can be ascertained, for example.
Grid strain can even be mitigated when excess electricity from EV batteries is used to power homes. “You’ve got bidirectional storage and discharge to the grid. You can potentially tap into the fleet of batteries that is growing over time to discharge electrons that might be stored when renewables are at their peak in order to smooth out some of that grid demand,” Wellise says.
Obstacles to AI Grid Optimization
While AI is taking great strides in making the grid more efficient, it has a way to go before reaching peak performance. Human intervention and monitoring are still necessary for many of its functions. The risk of allowing automated programs to take full control of the grid is simply too high — they cannot account for every potential problem that arises. The programs also pose security risks as they incorporate data from consumers, potentially exposing it to attackers.
In large-scale applications, data still must be collected, standardized, and enriched with metadata for AI to fully leverage it.
“A lot of these companies still are just grappling with improving the quality of the data and then integrating the data in one place and being able to use it,” Chaudhuri confides. “Many of them are still in the early stages.”
The installation of a greater array of sensors on the grid will allow for more diverse and detailed data sets to be aggregated and analyzed. Some programs may need special training due to the technicality of the language employed, which makes it difficult for more generalized programs to manage the information.
Selling utilities on the idea of AI management, as appealing as it is on paper, has proven challenging in some cases. “Infrastructure in the utility space is metal. It’s iron in the ground. It’s building power plants. It’s building transformers,” Demeo says. “Investors and banks are very comfortable with that. It’s very clear what that ROI is. Software seems unreliable compared to something you can actually see and physically touch and understand.”