What is Qualitative Data? | Definition from TechTarget
Qualitative data is descriptive information that focuses on concepts and characteristics, rather than numbers and statistics. The data cannot be counted, measured or expressed numerically. Researchers collect qualitative data from text, images, audio and video files, and other sources. After collecting the data, they analyze it and share it through data visualization tools, such as word clouds, timelines, graph databases and infographics.
Qualitative data analysis tries to answer questions about why people behave as they do and what motivates that behavior. Qualitative analysis is more time-consuming than other types of analysis because it takes longer to collect, analyze and present the data in a meaningful way. Someone who works with qualitative data is called a qualitative researcher or qualitative analyst.
Qualitative data analytics software (QDAS) is used in many research fields, including healthcare, education, social services, market research and other disciplines. In statistics, qualitative data is known as categorical data.
Types of qualitative data
Qualitative data is classified in different ways, depending on the needs and preferences of the researchers. One common approach is to categorize the data as either nominal or ordinal:
- Nominal. Nominal data is categorical data that cannot be ranked or ordered. The data is distinguished solely by its labels. For example, a dataset might include a category for eye colors, with values such as green, gray, brown, blue, amber and hazel. The colors alone identify the data elements.
- Ordinal. Ordinal data is categorical data that can be ranked or ordered in a related way. The data forms a hierarchy that establishes the relationship between the data. However, the relative differences between the hierarchy’s levels cannot be measured. For example, a category of school grades might include A, B, C, D and F, but there’s no way of knowing if D is twice as bad as C or F is twice as bad as D.
Some resources also include binary as a type of qualitative data. Binary data takes only two distinct values, such as on and off, yes and no, or positive and negative. Binary data is essentially nominal data that’s limited to the two values.
Qualitative data collection methods
Qualitative data is often collected through direct or indirect observation or by asking open-ended questions. Popular data collection methodologies include the following:
- Interviews. Interviews are one of the most common methods used to collect data. An interview can be as informal or structured as the situation warrants. It is often useful to ask open-ended questions, while letting the conversation flow naturally.
- Focus groups. A small group of individuals (usually six to eight people) meet to discuss a particular topic. The members of the group often share something in common, such as a similar experience, interest or skill. A moderator helps to control and direct the discussion.
- Surveys. Another common data collection technique is the survey, which enables researchers to reach a larger group of people than a focus group. Surveys might also be preferable to interviews or focus groups because respondents feel less intimidated by the process and more willing to open up.
- Longitudinal studies. Data is collected repeatedly from the participants over an extended period, with the focus on a specific characteristic or condition as it evolves over time. For example, researchers might use longitudinal research to study how patients have responded to a specific treatment over the long term.
- Records analysis. Researchers use reliable data that already exists to study a particular topic. The researchers use data mining techniques to uncover patterns and themes that help reveal the hows and whys hidden beneath the data.
- Observation. Researchers observe the study’s participants in their natural settings, noting how they respond to certain events. The researchers might also make video or audio recordings. Direct observation (sometimes referred to as shoulder surfing) enables them to gain first-hand knowledge of the subjects’ behavior without them needing to participate in interviews or responding to surveys.
- Case studies. Researchers conduct an in-depth investigation into a particular phenomenon. The investigation might target an individual or group in relation to their environment. The researchers learn how the subjects operate and behave within that environment, as it pertains to the phenomena being studied. The researchers might look at specific activities, situations or other factors to better understand the different dimensions.
What is qualitative vs. quantitative data?
Quantitative data is always numerical. It can be put in a database and analyzed using mathematical and statistical analysis methodologies. For this reason, the data is commonly structured. In comparison, qualitative data is descriptive and interpretive. It is based on language and concepts rather than on numbers, and it is typically unstructured.
The information gained from performing both quantitative and qualitative data analysis can be complementary. However, the goals for examining each type of data and the tools used to achieve those goals are different.
A quantitative data analyst seeks to answer objective questions about an event, such as how many or how often. In contrast, a qualitative analyst tries to answer subjective questions about the meaning that people assign to the event.
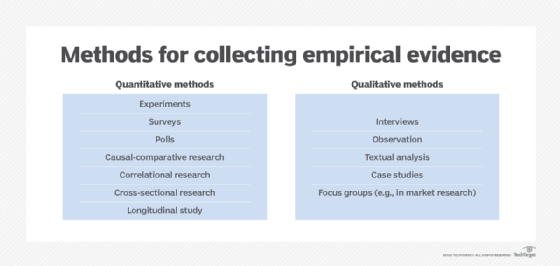
For example, a quantitative researcher might try to understand customer behavior by looking at what products a group of customers bought, when they made their purchases, where they made those purchases and how much they paid.
In contrast, a qualitative researcher might seek to understand the behavior of a subgroup of those customers by examining who they are in relation to the entire population, how they researched their purchases and why they made the decision to purchase one brand over the other.
Qualitative and quantitative data as complements
Researchers often employ qualitative and quantitative approaches together. They can operate in tandem with each other, one helping to inform the other.
For example, researchers who are studying an organization’s sales and marketing practices might first gather qualitative data to look at the customers’ purchase histories. From there, the researchers will gather quantitative data by interviewing the most loyal customers, as determined by the purchase histories. The researchers will then analyze the interviews to better understand how and why those people have been loyal customers.
From their analysis, they might realize that the majority of the interviews reflect the theme of superior customer service. From this realization, the researchers might go back into data from the company’s customer service platform and pull the response times by customers, as well as a measure of their brand loyalty. They might then be able to correlate fast response times with higher loyalty, thus establishing that fast response time is a key factor in establishing and maintaining loyal customers.
Pros and cons of qualitative data
Observational and content analysis methods help researchers avoid the Hawthorne effect, in which research participants modify their behavior because they’re participating in a study. Instead, researchers can collect data that more accurately reflects the actual circumstances. Qualitative data also adds color to a report and enhances the story being told through quantitative data alone, bringing generalized conclusions into a less abstract view, with real examples from real people.
However, collecting and analyzing qualitative data is very time-consuming. For this reason, researchers tend to use data sampling in their analysis, which makes it easier to collect and analyze the data. Unfortunately, small samples can make it difficult to scale out the results accurately enough to infer useful patterns. Quantitative analysis is much easier to carry out at scale.
Quantitative data can also be added directly into a database, and analysts can use mathematical formulas and numerical calculations to analyze it. In contrast, qualitative data must be categorized through descriptive parameters, such as traits or physical characteristics, before it can be statistically examined for patterns or meaning.
While quantitative data analysis can easily be performed by citizen analysts using software tools like spreadsheets, qualitative data analysis relies on the researcher’s skills and experience to create parameters from a small sampling through which larger data sets can be examined.
Qualitative data analysis
In qualitative data analysis, coding is used to categorize the data. Coding enables researchers to organize qualitative data in a meaningful way, using parameters that align with their research goals. Researchers also use a process called grounding the data, in which they assign codes to repeated patterns and then group the codes into a hierarchy to prepare them for statistical analysis.
In many cases, the coding process is recursive, rather than linear. For example, researchers might examine the data and identify a high-level theme, like customer satisfaction. As they dig further into the data, they will then subdivide it into subthemes, such as positive customer satisfaction and negative customer satisfaction.
Coding is part of the larger qualitative analysis process, which is generally based on one of the following two approaches:
- Deductive analysis. The analyst begins with a question and examines the data subjectively within the context of the question. Deductive analysis relies on predetermined codes that might come from existing frameworks, literature reviews, theoretical principles or other sources. The deductive approach is equivalent to using the scientific method.
- Inductive analysis. With inductive analysis, researchers start with a blank slate and let the codes emerge organically from the data. They carefully examine the data, looking for themes to appear as they process the information — e.g., read through transcripts or listen to recordings. An inductive approach generally takes longer than a deductive one. It requires many passes through the data to identify and refine the salient themes.
A frequent inductive method is called grounded theory. This simply means researchers are grounded in the data they have in front of them, rather than approaching the data with a preexisting theory or research question in mind.
Researchers also use different types of frameworks when conducting qualitative analysis. Two of the most common frameworks are Porter’s five forces and SWOT analysis (strengths, weaknesses, opportunities and threats analysis):
- Porter’s five forces. This framework was developed by Harvard professor Michael E. Porter to improve SWOT analysis. It identifies and analyzes the internal and external factors that can have an impact on success.
- SWOT analysis. Researchers used this framework to identify and analyze the internal and external factors that can have an impact on the viability of a project, product, place or person. SWOT analysis is useful to provide a snapshot for understanding the qualitative dynamics that affect success.
Sharing qualitative analysis
Conclusions drawn from quantitative data analysis are easily understood through the universal framework of mathematics. However, researchers working with qualitative data must be more creative in sharing their findings. Popular approaches to sharing qualitative data include:
- Word clouds. These data visualizations emphasize or deemphasize the frequency of various outcomes in a data sample.
- Timelines. Graphical representations such as timelines indicate significant events or milestones in a data sample in relationship to the passage of time.
- Graph databases. These are databases that graphically illustrate the relationships between data elements. Like word clouds, graph databases can make qualitative data easier to understand through the use of size, color and placement to emphasize or deemphasize the value of data elements within the sample. Graph databases are also referred to as concept maps.
- Infographics. An infographic uses charts, illustrations and minimal text to present findings visually in a story form that can be easily understood.
Traditional quantitative visualizations, such as histograms or bar charts, are also frequently used once coding has been completed and the frequency counts established for the tags or themes. In some cases, qualitative data is included to support conclusions from quantitative analysis. The qualitative data might come in the form of an example, quote, clip, callout or other format.
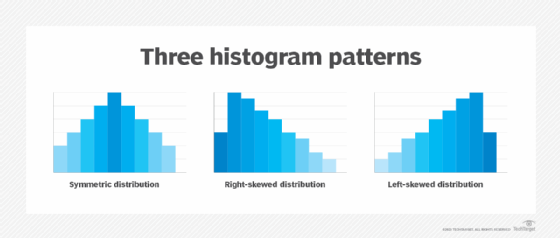
Qualitative data analysis tools
Researchers commonly use qualitative data analysis software (QDAS) to carry out their analysis. The software helps to shorten the time it takes to collect and analyze qualitative data. Typically, QDAS includes features such as the following:
- Robust data management.
- Data coding and categorization.
- Text and content analysis.
- Support for multiple file types, including audio and video.
- Reporting and visualization.
Software programs for qualitative analysis were first marketed in the mid-1980s. Today, qualitative data analysis software is an established market. Key players according to experts include the following:
- ATLAS.ti.
- Dedoose.
- Dovetail.
- HyperResearch.
- MaxQDA.
- NVivo.
- QDA Miner.
- Quirkos.
- Raven’s Eye.
A data-driven decision-making framework provides guidelines that any organization or individual can use. Explore key steps from a data-driven decision-making framework.